Outliers in Machine Learning
Outliers in Machine Learning, Are you the one who is looking forward to knowing Outlier detection introduction in Machine Learning? Or the one who is looking forward to knowing outlier detection techniques in Machine Learning and the effects of outliers in data or Are you dreaming to become to certified Pro Machine Learning Engineer or Data Scientist, then stop just dreaming, get your Data Science certification course with Machine Learning from India’s Leading Data Science training institute. Outliers are data points that are distant from other similar points due to variability in the measurement. Outliers should be excluded from the data set but detecting of those outliers is very difficult which is not always possible. The below blog clearly explains your effects of outliers in data and how to identify outliers in data. Do you want to know outlier detection introduction, So follow the below-mentioned outliers in the machine learning tutorial from Prwatech and take advanced Data Science training with Machine Learning like a pro from today itself under 10+ Years of hands-on experienced Professionals.Outlier Detection Introduction
A data point that lies outside the overall distribution of the dataset is called an outlier of the data. An outlier is an observation point that is distant from other observations statistically, i.e. Outlier is separate or different from a point or set of points in the group. So in short we can call it as ‘odd man out’ from the datasetEffects of Outliers in data:
Due to variability in data or experimental errors like mistakes in data collection, recording, and entry, the datasets contain outliers. In the case of statistical analysis, it can cause major problems like :Data skewing
Errors in the mean of the data set.
Errors in the standard deviation of the data set.
How to Identify Outliers in Data?
To identify outliers effectively, we can employ two approaches within the framework. DIFFERENT TYPES OF OUTLIERS: In various datasets, we encounter two distinct types of outliers that require specific handling.- Uni variate (one variable outlier): This involves analyzing outliers within a single variable.
- Multivariate outliers (Two or more variable outliers): This pertains to scenarios where we assess outliers across multiple variables, particularly when one of them is categorical.
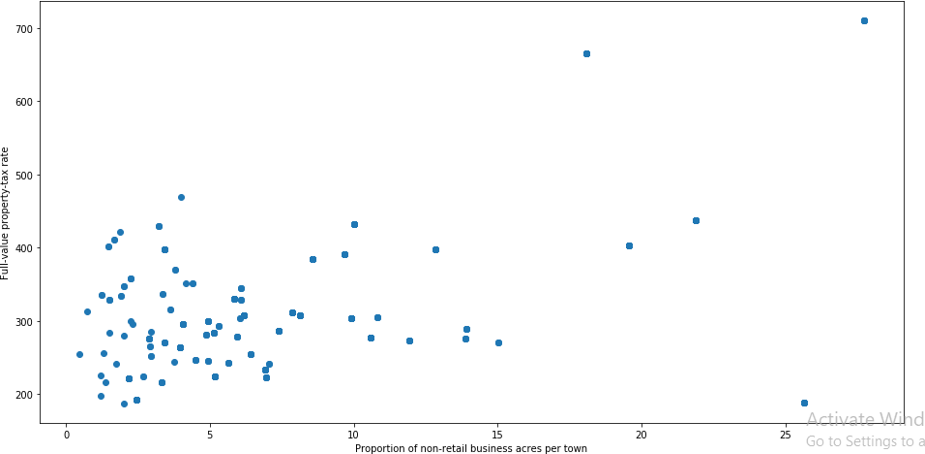
Using Box Plots:
A box plot is a method for representing collections of numerical data through their quartiles. Outliers may be plotted as individual points in this graphical representation. So from this, we can find out the separately placed points in the box plot as outliers. First load the boston file from sklearn: import pandas as pd import numpy as np from sklearn.datasets import load_boston boston = load_boston() x = boston.data y = boston.target columns = boston.feature_names #create the data frame with x and y df = pd.DataFrame(boston.data) df.columns = columns df.head() Now we will plot the box plot using ‘box plot’ import seaborn as sns sns.boxplot(x=df['DIS']) We get the box plot as: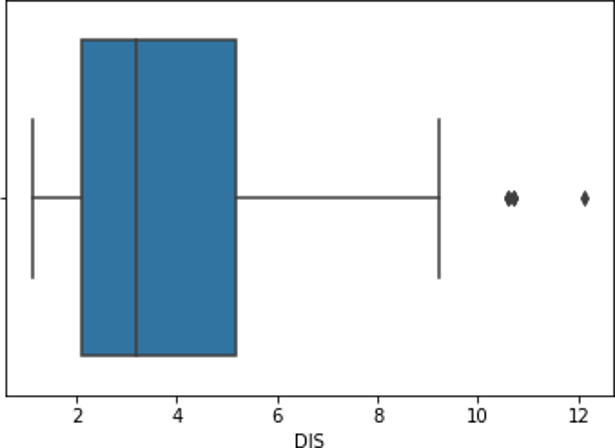
Finding Outliers with Mathematical Function
Using Z-score:
Z-score is used to describe any data point by finding their relationship with the Standard Deviation of the dataset and the Mean of the group of data points. Z-score is identifying the normal distribution of data where the mean is 0 and the standard deviation is 1.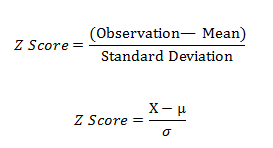